What are Privacy Enhancing Technologies?
Privacy Enhancing Technologies (PETs) enable businesses to obtain valuable insights from data while ensuring personal data protection, data privacy, and the safeguard of commercially sensitive information. PETs allow for increased B2B data collaboration, cross-border data flow, and data collection for AI development.
IMDA’s PET Sandbox: A safe space to trial PETs
As PETs are still in their infancy, there is much to learn about using these technologies in a real-world environment. To facilitate experimentation with PETs, IMDA’s PET Sandbox – Singapore’s first – provides opportunities for companies to work with trusted PET digital solution providers to develop use cases and pilot PETs.
The PET Sandbox will:
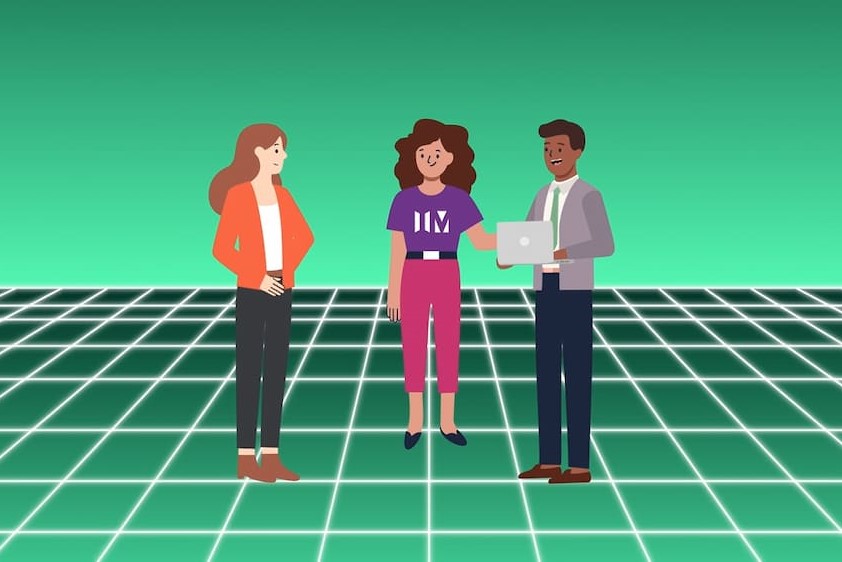
The PETs sandbox provides a testing ground for businesses to pilot their PETs use cases, with technology, financial, and regulatory support from IMDA, across three archetypes: (a) identifying common customers in multiple datasets; (b) deriving additional features of common customers from multiple datasets; and (c) making more data available for AI.
New archetype for PETs Sandbox: Data use for Gen AI
Recognising that PETs holds the same potential for generative AI use cases, IMDA has expanded the PETs Sandbox to support projects belonging to the fourth archetype “Data Use for Gen AI” – explore how PETs can be used to unlock more data for generative AI use cases while addressing data protection related risks. This new archetypes will focusing on data use model and application development and use.
Through the PETs Sandbox, PDPC has launched the Guide on Synthetic Data Generation to help organisations understand the synthetic data generation techniques and its potential use, particularly for AI.
Case studies
Since the launch of the PET Sandbox, IMDA has received active participation from the industry. Read about the PET use cases implemented by the participating businesses:
Customer engagement and experience can be enhanced by offering relevant promotions. This can be predicted using a model that is trained using preference and behavioral data that is collected by different data partners. In this POC, Ant and partner explores training a more accurate prediction model by using preference and behavioral data from their partners, without revealing sensitive information regarding their customers or business during the entire process.
Learn how to enhance customer engagement with privacy preserving AI (1.45MB).
GPAI and IMDA collaborated to demonstrate how privacy-enhancing technologies can be used to share data from past pandemics and improve societal resilience to future outbreaks. This report shares key findings from this project, highlighting the importance of circulating data via confidential and trustworthy channels.
Learn how trustworthy privacy-enhancing technologies can be used to overcome data barriers (1.41MB).
Faster market and data-driven decision making can be enabled by faster access to data. In this POC, Grab transformed their manual data tagging and clearance process to one that is automated with LLM based metadata tagging and automated data anonymisation to provide quick data access without compromising on personal data.
Learn how to enable data availability through automated data management (612.18KB).
Data sharing amongst the pharmaceutical company and its data partners has not been easy as they have to abide to the different data protection regulations in various jurisdictions. Often these data involves individuals and may disclose product distribution and transaction data within the ecosystem players. The pharmaceutical company designed a Trusted Execution Environment (TEE) based solution for the POC, where it included safeguards to ensure that the original data from data partners cannot be read, modified, or accessed in any form by host of the environment. Through the POC, the company managed to access more data from its data partners and create new data models that benefits the ecosystem partners.
Mastercard, a global technology company in the payments industry, via its Cyber and Intelligence Solutions business line has been assessing the potential for frontier technologies, including Privacy Enhancing Technologies (PETs), to buttress its product offerings against financial crimes like money laundering.
Mastercard developed a proof of concept (POC) in IMDA’s PET Sandbox program to investigate a product based on Fully Homomorphic Encryption (FHE), provided by a third-party supplier, for sharing financial crime intelligence across international borders – specifically between Singapore, the United States (“US”), India and the United Kingdom (“UK”) – while complying with prevailing regulations.
Tracking technologies, like 3rd party cookies, are presently a mainstay in the digital advertising ecosystem. Publishers, advertisers and adtech firms rely on the collection and sharing of user / device identifiers to analyse how consumers can be shown advertisements best aligned to their online interests or activities.
However, the ecosystem is preparing for a paradigm in which the collection of user / device identifiers that are linkable across apps / websites is no longer feasible, and trust in the ecosystem is low. A prominent avenue where solutions to measure attribution of digital ads without tracking technologies is actively discussed is the World Wide Web Consortium (W3C)’s Private Advertising Technology Community Group, or “PAT-CG”.
Meta and Mozilla, as members of PAT-CG, have proposed a solution – “Interoperable Private Attribution” or IPA. It uses a combination of multiparty computing (MPC), aggregation, differential privacy (DP) and write-only identifiers to enable attribution measurement. The solution aims to measure advertising outcomes based on impressions shown on publisher website(s)/app(s) and conversions occurring on an advertiser website/app.
Find out how Meta and its partners piloted ‘Interoperable Private Attribution’ (439.36KB), a PET-based solution to generate attribution reports without use of 3rd party cookies.
For more insights into PETs, please access the following reports:
IMDA-Google: PET x Privacy Sandbox
IMDA has partnered with Google to give companies access to Google’s Privacy Sandbox through IMDA’s PET Sandbox environment. Key features of the PET x Privacy Sandbox include:
Find out more about the PET x Privacy Sandbox here.
Contact us
For queries, please email to data_innovation@imda.gov.sg